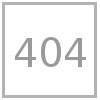
The main interest of the team is the inference and exploitation of molecular networks to extract information from complex omics datasets. We develop methods and apply them in collaboration with biologists and clinicians to the analysis of small, specific tumor cohorts. A strong emphasis is put on the tumor microenvironment and its cellular networks, which we investigate using bulk, single-cell, or spatially resolved data. We are starting to apply these techniques to autoimmune diseases. Network science and machine learning (ML)/AI are the preferred tools for this type of study.
We also develop methods related biomarker discovery. In the field of cancer research, we mainly work on circulating DNA and fragmentomics data using ML/AI. We also employ numerical analysis and Bayesian approaches to model mass spectrometry data and protein turnover in vivo in Alzheimer disease searching for abnormal dynamics.
Axis 1: Molecular network inference (Lead: Ravel & Colinge)
The analysis of large-scale (multi-)omics datasets is often difficult. Contextualizing data using molecular interaction networks facilitates the extraction of reliable information. We have developed a unique framework to infer cellular networks combined with intracellular pathways. Such analyses can be conducted from bulk, single-cell and spatial data alike (Cabello-Aguilar, NAR, 2020; Villemin, NAR, 2023), which we keep extending with AI techniques notably.
We also proposed a new family of statistical models to infer molecular interactions from perturbation data (Borg, Bioinformatics 2023 & bioRxiv 2024). Generalization of this theory is an active topic.
Axis 2: Biomarker discovery through mathematical modeling (Lead: Colinge)
Applying numerical analysis and ML/AI methodology, we are engaged in various biomarker discovery research programs. A first example concerns the search for abnormal protein dynamics in Alzheimer disease (Lehmann, Anal Chem, 2019; Giroux, J Proteome Res, 2024; Lehmann, Bioinformatics, 2024).
Another example is circulating DNA in cancer detection (Tanos, Adv Sci, 2020). Furthermore, we applied notions of spatial statistics and ML to investigate tumor stiffness spatial heterogeneity relation with clinical parameters (Lopez-Crapez, Sci Rep, 2022), or classical ML to characterize the tumoral microenvironment (Benhamouda, Clin Cancer Res, 2022).
Axis 3: Applications of systems biology (Lead: Cornillot & Colinge)
The development and the application of advanced computational methodologies related to functional genomics leads to recurrent collaborations with biologists and clinicians. Typical studies in this category consist of small- to medium-sized tumor cohorts or specific biological models (Alame, Theranostics, 2020 & 2021; Giguelay, Theranostics, 2022; Souche, Endoscopy, 2021; Honda, Theranostics, 2024; Figarol, Nat Comm, 2024; Singh, Nat Microbiol, 2023; Rabia, Front Immunol, 2023). We typically combine data integration with methods of the axes 1 & 2 or existing tools by others.